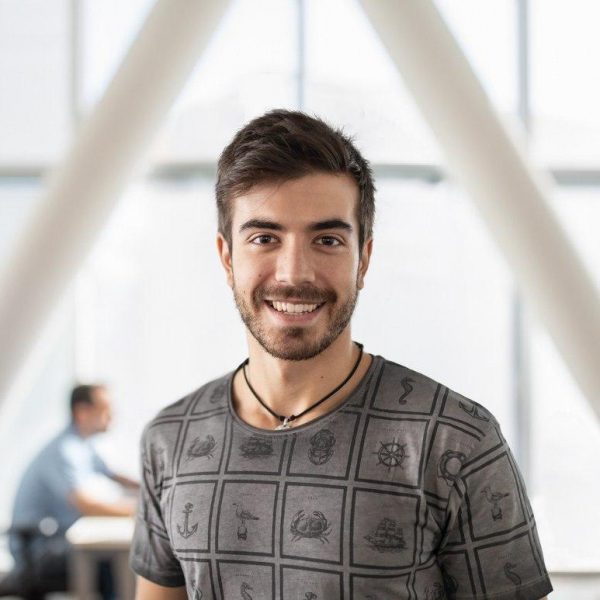
Early Stage Researcher at UOM
Michele has a background in Computer Science and Machine Learning, he has been working in generative language model applied to journalism. Now he is working on generative multimodal models with a focus on model transparency and semantic grounding. He enjoys running, sports and the outdoors.
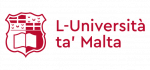
Institute of Linguistics and Language Technology,
University of Malta (UOM)
Tal-Qroqq, Msida, MSD2080, Malta
Connect
- +39 366 330 7776
- michele.cafagna@um.edu.mt
Follow
Multimodal Semantic Grounding and Model Transparency
PhD research topic
· Main Supervisor: Dr. Albert Gatt, Institute of Linguistics and Language Technology – Università ta’ Malta (UOM), albert.gatt@um.edu.mt
· PhD Co-Supervisor: Prof. Kees van Deemter, Department of Information and Computing Sciences- Universiteit Utrecht (UU)
· Inter-sectoral Secondment Supervisor: Dr. Lina María Rojas Barahona, Learning and Natural Dialogue Teams – Orange
· Inter-sectoral Secondment Supervisor: Dr. Johannes Heinecke, Learning and Natural Dialogue Teams – Orange
Objectives:
– To develop methods for evaluating the sensitivity of neural generation models to their input, extending current techniques for sensitivity analysis;
– to develop new multimodal architectures based on an in-depth exploration of the optimal visual features for NLG tasks such as the generation of descriptive or inferred text from images or videos. While several architectures have been proposed at the Vision-Language interface, it is often observed that generation from visual input suffers from a lack of sensitivity to the input, which may be due to problems in the multimodal representations learned by the architecture, and/or issues of redundancy and preedictability in the trainning data;
– to design training setups that carefully control for bias and predictability in training data and in the output.
Main challenge
Our main challenge will be to study deep multimodal architectures for generating language from visual data, looking ‘under the hood’ for evidence that the choices made by a generator are indeed grounded in the input, that is, motivated by (and explainable on the basis of) the sensory information.
Expected Results:
Thus, the expected outcomes are:
(1) In-depth theoretical and practical understanding of neural network methods and the underlying theoretical basis of multimodal neural architectures.
(2) To develop sensitivity analysis tools for NLG in general, and especially for NLG from visual input, which gobeyond the state of the art.
(3) Implementation of diverse multimodal neural architectures.
(4) New dataset for vision-text tasks (e.g., image captioning, grounded textual entailment generation) with an emphasis on high diversity and sensitivity of textual samples to visual inputs, and with an attention to design issues to avoid redundancy between modalities.
(5) Design and execution of evaluation experiments, both automatic and involving human participants, to evaluate sensitivity and the groundedness of multimodal models.