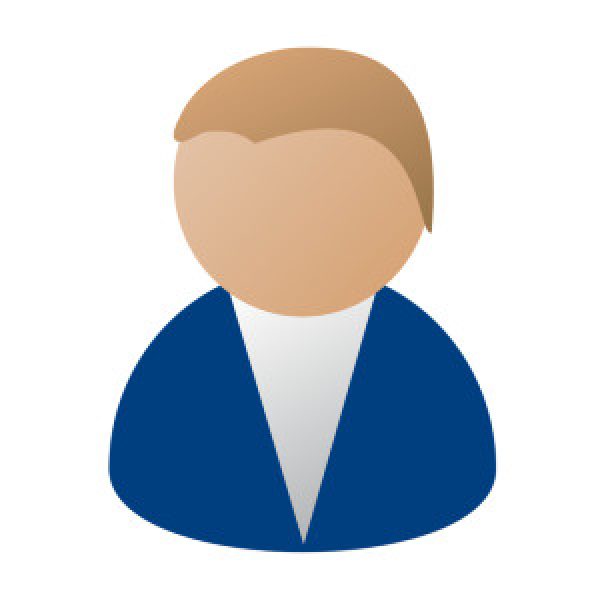
Early Stage Researcher at IIIA-CSIC
Qurat-ul-ain has a background in Artificial Intelligence and dependable software systems. She is interested in interactive intelligent systems and decision support systems. She is passionate about making AI explainable, ethical and accessible to people from all walks of life. She enjoys reading, cooking and exploring other cultures.
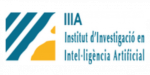
Artificial Intelligence Research Institute (IIIA)
Agencia Estatal del Consejo Superior de Investigaciones Científicas (CSIC)
Carrer de Can Planes, Campus UAB
08193, Bellaterra, Catalonia, Spain
Connect
- +34 641 6969 74
- qurat@iiia.csic.es
Follow
Argumentation-based multi-agent recommender system
· Main Supervisor: Prof. Dr. Carles Sierra, Artificial Intelligence Research Institute (IIIACSIC) – Agencia Estatal del Consejo Superior de Investigaciones Científicas (CSIC), sierra@iiia.csic.es
· Main Supervisor: Prof. Dr. R. López de Mántaras, Artificial Intelligence Research Institute (IIIACSIC) – Agencia Estatal del Consejo Superior de Investigaciones Científicas (CSIC)
· PhD Co-Supervisor: Dr Katarzyna Budzynska, Instytut Filozofii i Socjologii Polskiej Akademii Nauk – Institute of Philosophy and Sociology of the Polish Academy of Sciences (IFIS PAN)
· Inter-sectoral Secondment Supervisor: Mr. R. Diñeiro, Accenture Technology
PhD research topic
Objectives:
This PhD thesis will explore an interactive distributed model in which recommenders become active software agents that accompany the recommendations with arguments. This approach is radically different with previous recommender system approaches (content-based or not) where the experiences of users are represented as passive data in a database. These recommender agents will be able then to engage in argumentative dialogues with other recommenders to agree (or not) on a recommendation to the user. A mediator may pair recommenders according to different criteria (e.g., similarity of profiles with the user, dissimilarity of their experiences) and then aggregate the outcomes of the different dialogues into a meaningful recommendation to the user that will be duly justified by arguments.
Expected Results:
(1) Initially, publicly available data sets will be used for validation of the approach, in particular, the Sushi data set73, which offers a well-designed source of preference data that can be used to form arguments for supporting recommendations.
(2) The approach will address two main issues still remaining in the recommender systems – the sensitivity of the precision of preference estimation with respect to the measurement error in the profile data; and the effect of central tendency effect (the tendency to select neutral positions on rating scales).
(3) A comparison with current implementations of content-based recommender systems will be done.
(4) At least two use cases will be identified where the results of the PhD work will be applied and evaluated.